DeepMind Say Reinforcement Learning is the Way to Mastering General AI
DeepMind in a new paper argue that reinforcement learning is the way to mastering general AI. In other words, it is not just the sum of all the individual decisions that matters, but their interplay that ultimately makes the difference.
In an effort to recreate true intelligence, computer scientists have designed and built the many different and complicated mechanisms required to simulate vision, language, reasoning, and motor skills. They also developed techniques capable of being applied over a limited environment. However, they have yet to recreate the type of general intelligence seen in human beings and dogs.
In a new paper submitted to the peer-reviewed Artificial Intelligence journal, scientists at the AI lab DeepMind argue that intelligence and its associated abilities will only emerge from primitive yet powerful principles such as ‘maximizing reward.’
The report, titled “Reward is Enough,” studied the evolution of living organisms to develop the kind of abilities often associated with intelligence. The researchers suggest that reward maximization and trial-and-error are enough for behavior to be developed. These findings suggest that reinforcement learning is a possible mechanism relating to the development of artificial general intelligence.
Twin paths for AI
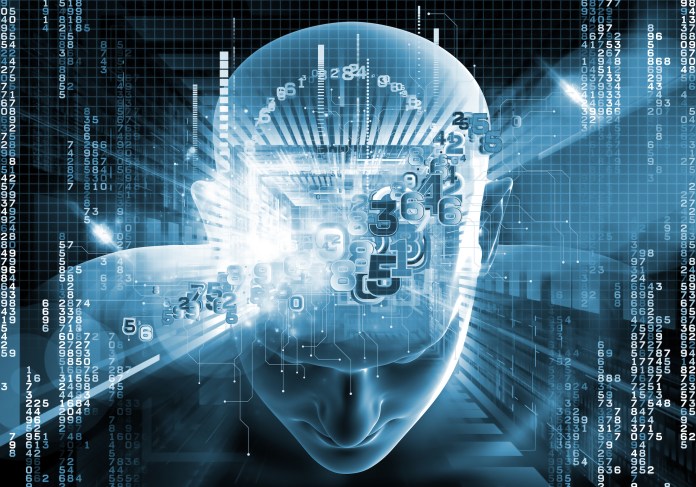
Improvements by design, feedback, and feature input are often used to improve the behavior of AI. To create AI, specialists often study animal-like intelligent behaviour in organisms. For example, understanding how a mammal’s vision system works has led to a wide variety of AI that categorizes images, locates objects in photographs and more. Likewise, our knowledge of language is being used to fund the development of various systems that are able to produce or answer questions. These include text generation and translation systems.
This is another aspect of narrow artificial intelligence, this is a system designed to perform only one certain tasks instead of it being able to general problem solving skills. It has been discovered that merging separate AI modules can produce a whole, more intelligent computer program. For example, you have a software program that coordinates between different sight, voice processing, linguistic and other written data processing modules to solve complicated tasks which require many skills.
Based on the DeepMind’s proposed idea, scientists are considering a different approach to creating artificial intelligence. It is “to recreate simple yet effective rules that have given rise to natural intelligence.” They “consider an alternative hypothesis: that the generic objective of maximizing reward is enough to drive behaviour that exhibits most if not all abilities that are studied in natural and artificial intelligence,” according to researchers.
For the most part, life continues to go about its business as though it has been a mindless formula. Nature has been successful in constructing us organisms that are heavily reliant on evolution, natural selection, good genes, and a sense of self preservation.
All living beings have different skills and abilities that help them amongst each other. One of the most significant mechanisms that frees them from predators is their ability to perceive and adapt to environments that they are navigating.
The DeepMind researchers argue that:
“The natural world faced by animals and humans, and presumably also the environments faced in the future by artificial agents, are inherently so complex that they require sophisticated abilities in order to succeed (for example, to survive) within those environments…Thus, success, as measured by maximising reward, demands a variety of abilities associated with intelligence. In such environments, any behaviour that maximises reward must necessarily exhibit those abilities. In this sense, the generic objective of reward maximization contains within it many or possibly even all the goals of intelligence.”
Take for example a squirrel who needs to get food. On the one hand, it uses its sensory and motor skills to find the nuts that are available when it is hungry enough. Although a squirrel that can only find food will die when the scarcities more of the time. They, the squirrels, have memory and planning skills to cache the nuts so they won’t be hungry again. The squirrel also has social skills that help them from getting their kernel from being taken by other animals. You could argue that staying alive is a whole objective in itself. That also involves knowing how to defend themselves against dangers that exist and finding a better place for them to go when they have season changes.
“When abilities associated with intelligence arise as solutions to a singular goal of reward maximisation, this may in fact provide a deeper understanding since it explains why such an ability arises,…In contrast, when each ability is understood as the solution to its own specialised goal, the why question is side-stepped in order to focus upon what that ability does.”
Finally, the researchers detail how things should happen in order to maximize reward. The most general and scalable way to do it is by interacting with the agent’s environment while they are going in order to maximize the reward.
Using reward maximization to develope abilities
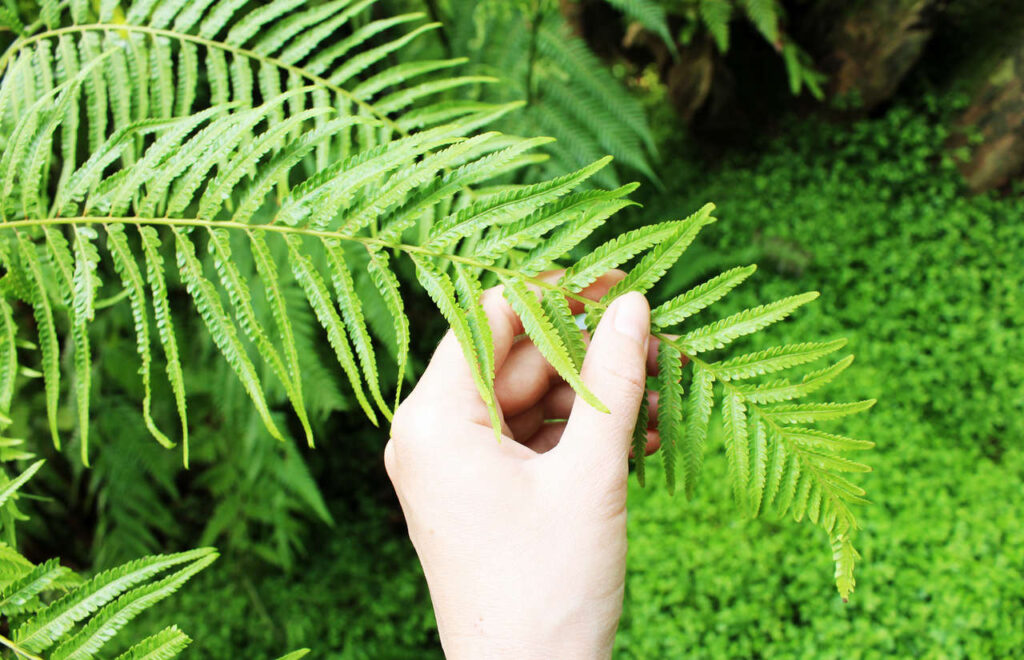
The researchers gave a few sophisticated examples of how
“intelligence and associated abilities will implicitly arise in the service of maximising one of many possible reward signals, corresponding to the many pragmatic goals towards which natural or artificial intelligence may be directed.”
One example argued how sensory skills serve the purpose to be in a complicated environment because of they can find food, prey, friends, and threats. Detection enables them to know a piece of fruit and avoid fatal options such as running off of a cliff. At the same time, ears let them find threats where they can’t see or get food when it is camouflaged. Touch, taste, and smell also help them experience habitats in better ways with no threats. This means they have a greater chance for their survival in dangerous environments.
Rewards and environments will influence the type of knowledge innate or learned for animals. For example, animals in a hostile habitat dominated by predator animals that was attacked often times in the past, such as lions and cheetahs, were rewarded for an innate knowledge to run away where they could find food and shelter. Meanwhile, rewards for other animal species is the ability of learned knowledge to find food and shelter in their habitat.
The researchers also discussed how language is reliant on the input and satisfaction of seeking joy, social intelligence, imitation, and finally general intelligence. They considered this one complex area and found the best course of action is to be always looking for the reward, “maximising a singular reward in a single, complex environment.”
Thus, they are suggesting that the natural intelligence similar to artificial intelligence. An animal’s list of experience is sufficiently rich and complex that they may need to have a flexible ability in order to complete goals for instance foraging, fighting, and escaping. In order to ultimately maximize what they are getting such as hunger or reproduction. So, if an agent’s experience has many different kinds of experience, then the goals of the agent should be the same to. It should have battery life and it should survive. The reward should be enough for an agent to have a positive result.
Reinforcement learning and reward maximization
Reinforcement learning falls within a branch of AI programs that include three key parts: an independent, agents, and rewards.
Performing an action will change the agent themselves and the environment. The more impactful the agent does, the more reward they will get. In many reinforcement learning problems, the agent does not know the environment at first. Based on how it is doing, the agent can learn to customize their actions for a higher reward.
The paper by the DeepMind group suggests reinforcement learning as the way to replicate reward maximization seen naturally by large numbers of species. Eventually, this could lead to artificial general intelligence.
“If an agent can continually adjust its behaviour so as to improve its cumulative reward, then any abilities that are repeatedly demanded by its environment must ultimately be produced in the agent’s behaviour,” the researchers write, adding that, an agent that is good at reinforcement learning would be able to get skills like perception, language, and social intelligence
In the article, the researchers provide various examples that demonstrate how reinforcement learning agents can acquire skills in games and in a robotic environments.
However, the researchers still haven’t found a way to solve the fundamental problems. For example, “We do not offer any theoretical guarantee on the data efficiency of reinforcement learning agents.” Although, Reinforcement learning is notoriously better at finding the correct answers through comparing the data than without it. For example, a reinforcement learning agent may need centuries of gameplay spaces to master a computer game. And AI researchers haven’t figured out how to create reinforcement learning systems that can generalize too many domains. Therefore, a model or machine might need to have the whole training when the environment is changed.
The researchers have also noted that learning mechanisms for reward maximization is still a question that needs to be further solved.
Reward maximization: strengths and weaknesses
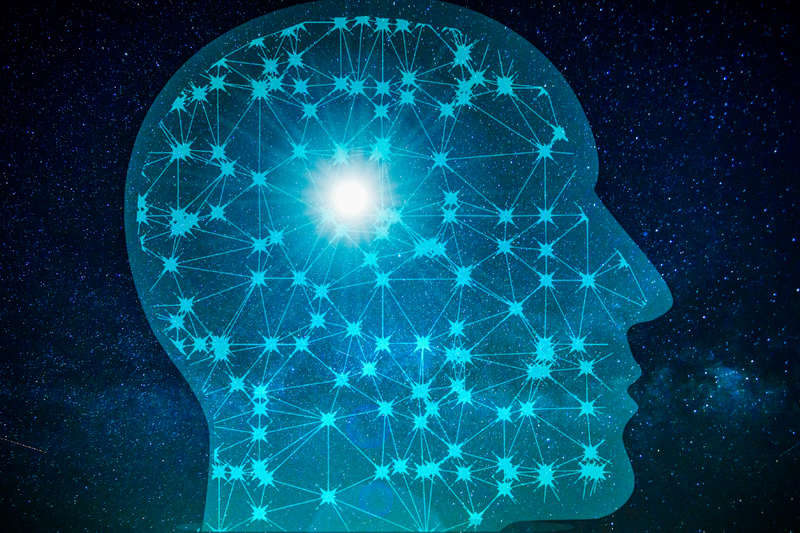
Neuroscientist, Patricia Churchland, who is Professor Emerita at the University of California argued that the ideas in the paper were “very carefully and insightfully worked out.”
Churchland pointed out that the paper might have shortcomings. The DeepMind researchers focus on personal gains in social interactions. Regarding this topic, Churchland, who has recently written a book on the biological origins of moral intuitions, argues that attachment and bonding has a powerful influence in the social decision-making of mammals and birds, which is why animal put themselves in danger to protect their young.
“I have tended to see bonding, and hence other-care, as an extension of the ambit of what counts as oneself—‘me-and-mine,’” Churchland said. “In that case, a small modification to the [paper’s] hypothesis to allow for reward maximization to me-and-mine would work quite nicely, I think. Of course, we social animals have degrees of attachment—super strong to offspring, very strong to mates and kin, strong to friends and acquaintances etc., and the strength of types of attachments can vary depending on environment, and also on developmental stage.”
Herbert Roitblat a renowned Data Scientist and author of Algorithms are Not Enough argued that as it blurs the lines between humans and dumb animals. It appears to claim that simple mechanisms and trial-and-error can teach animals skills like those associated with intelligence. Roitblat went to point out the theories postulated in the paper can be difficult to implement in real life
“If there are no time constraints, then trial and error learning might be enough, but otherwise we have the problem of an infinite number of monkeys typing for an infinite amount of time,” Roitblat said.
A lot of interesting ideas have been presented in the paper and it lays down possible paths for A.I to develop along. The one thing that is for certain is that the next few years are going to be very exciting in terms of seeing where A.I. goes.

Staff writer. Jonas has an extensive background in AI, Jonas covers cloud computing, big data, and distributed computing. He is also interested in the intersection of these areas with security and privacy. As an ardent gamer reporting on the latest cross platform innovations and releases comes as second nature.